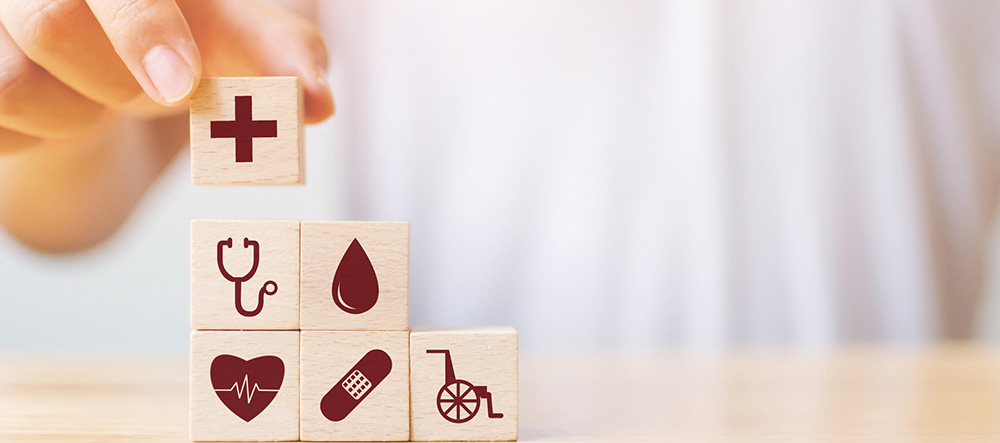
26 Feb Natural Language Processing (NLP) Driving SDOH Adoption
While social determinants of health (SDOH) efforts have risen in importance for payers and providers, there are still barriers to full adoption.
According to a recent Health IT Analytics article, collecting patient data and ensuring that all information is representative of whole populations remains a challenge. In addition, many providers lack the time and resources to draw meaningful, actionable conclusions from social determinants data.
The article highlighted a 2017 study from Massachusetts General Hospital (MGH) that pointed to how natural language processing (NLP) techniques could help extract meaningful socioeconomic data in the EHR and predict psychosocial risk.
For example, since SDOH information is usually collected within the EHR in an unstructured format, NLP solutions can help providers obtain the SDOH information needed to refer patients to critical social services.
NLP is ideal because of the ongoing pressure to leverage increasing amounts of validated patient data, which is another reason why providers are seeking ways to identify concepts, context and codify unstructured text based data.
Fortunately, there are industry solutions for meeting these needs.
By using best-of-breed NLP technologies, IMAT transforms and annotates unstructured medical narratives – such as those found in medical records or dictated clinical summaries – and turns them into actionable data.
This provides the opportunity to leverage untapped information for code mapping, concept/context identification, and developing standard/custom dictionaries.
If you would like to learn more about how the IMAT’s Natural Language Processing capabilities can help you leverage unstructured patient data, please contact us.
Sorry, the comment form is closed at this time.